In addition to the corpus research, it selected a few cases to assess the machine translation quality from a qualitative perspective. Major findings of this study are listed in the first section. Although the present study has come to a preliminary conclusion that translation machine performance on literary text is unsatisfactory, limitations still remain. Given that more studies may be carried out on this subject in the future, suggestions are offered in the last section of this chapter. This study found answers to the three research questions raised in the beginning: (1) Is there any significant positive correlation in three linguistic indicators between literary text and its machine translation? There is a significant positive correlation on TTR between literary text and its machine translation while no significant correlation is found on either Average Sentence Length or Content Words Density. (2) Is there any significant positive correlation in three linguistic indicators between non-literary text and its machine translation? Significant positive correlation is found on all three indicators, namely TTR, Average Sentence Length and Content Words Density, between non-literary text and its machine translation.
.......
1Introduction
Translators react to the coming wave of AI technology with a mixed feeling of hope and concern. On the one hand, translators across all fields take advantage of many newly developed technological tools like online dictionaries, translational corpus and translation memory software that make their work more agreeable and effective and thus “release their cognitive resources for complex tasks by relieving them of repetitive and boring tasks” (Taivalkoski-Shilov, 2018). On the other hand, the threat of replacement now not only comes from other professional translators as in the past, but also from raw or post-edited machine translations which in clients’ view represent cheaper price and shorter delivery time. To explore how translators feel about machine translation, a research group from Zurich employed automatic sentiment analysis on a large collection of online posts (from Facebook, Linked In and Twitter) about translators’ opinions on machine translation, and the experimental evidence shows that negative perceptions in social media outnumber positives (Läubli & Orrego-Carmona, 2017). After studying a full range of translation cases in market, Way (2013) proposed a rule of thumb that the degree of human involvement in translation scenario should be associated with the lifespan of the content, and claimed that “those translators who argue that there is only one level of quality, namely ‘flawless’ human translation, are obviously stuck in the dark ages”. In other words, it is moot to question whether machine translation is useful or not, and the focus now is to distinguish which types of source texts are suitable for using machine translation and which still rely on human translators. When it comes to which type of texts shall still rely on human translators, the first one must be literary works, which generally represent the wisdom collections of human beings passing down through generations. In fact, “no one seriously believes that a computer could understand Shakespeare since the intuitions of most people, both in and outside academia, are that computers are too rigid, formulaic, and cold to pick up the subtle nuances, emotions, and creative brilliance manifested in literature” (Graesser et al., 2011). Although the proposal that machine understands literature would be blasphemous for readers and translators who focus on literary works, it is undeniable that machine translation might one day rise to the human intelligence level. Hence one essential task facing both developers and translators is to always follow up machine translation performance on literary works. Only by knowing how far machines have achieved on this translation type can researchers timely update their research direction and translators better plan for the future.
......
2.Literature Review
2.1Literature Review on Machine Translation
The term Machine Translation refers to computerized systems producing translations of oral or written texts in different languages. The ideal goal of machine translation is to automatically produce high-quality translations without any human assistance. As Hutchins (1995) concluded at the start of Machine Translation: A Brief History, “The translation of natural languages by machine, first dreamt of in the seventeenth century, has become a reality in the late twentieth”. Before the landing of functioning translation machines was a longtime exploratory stage. In 1933, George Artsrouni invented a storage device similar to a multilingual mechanical dictionary, through which equivalent words of other languages could be found to facilitate human translation and in the same year Petr Smirnov-Troyanskii put forward a systematical idea of how to achieve easier translation in a mechanical way (Hutchins, 2007). Later in 1951, Yehoshua Bar-Hillel, a linguistic researcher at the Massachusetts Institute of Technology (MIT), started to devote his time on machine translation realization and convened the first machine translation conference on which one important idea that although perfect machine translation was a virtual impossibility, pre-editing or post-editing was expectable took shape (Hutchins, 2007). The fast-developing machine translation does bring great benefits to users by improving translation speed or reducing translation cost, while at the same time it causes a lot of concerns and thus will not replace human beings for the foreseeable future. Taivalkoski-Shilov (2018) reminded of ethical issues regarding machine translation of literary texts which might lead to low quality and unnecessary noises. The popularity of machine translation may interfere with the actual language acquisition process or even reduce the desire to learn a second language (Groves & Mundt, 2015). Prates et al. (2018) noticed that there was a growing concern about the phenomenon dubbed as machine bias which meant that trained statistical models reflect gender or racial bias, so they conducted a study that “built sentences in constructions like ‘He/She is an Engineer’ (where ‘Engineer’ is replaced by the job position of interest) in 12 different gender neutral languages such as Hungarian, Chinese, Yoruba, and several other” and then translated these sentences into English in Google Translate, finding that translation tools such as Google Translate showed gender biases and a strong inclination for male defaults. To sum up, the development of automated translation will not result in the passivation and exclusion of human beings, since translation machines are artifacts based on human creativity and require lifelong learning from human beings.
2.2Literature Review on Literary Translation
Many scholars put their focus on literary translation studies and propose many literary translation theories, trying to find the best way to keep the original beauty of literary works. Many golden rules proposed by famous translation theorists like Eugene A. Nida and Peter Newmark are applied into practice in literary translation, and translation theories exclusively belonging to literature works also emerge in endlessly. Professor Lefevere (2016) whose major research field was in translation and comparative literature study once proposed “Rewriting Theory” to guide literary translation. Kazakova (2015) suggested that in dealing with literary translation which was a specific type with sophisticated structures of information, effective strategies of bilingual information processing including observer-strategy, helper-strategy and enlightener-strategy were needed as attempts to manage the complications. It is known to all that how to translate literary works has always been a difficulty and the crux lies in the cultural differences, starting from which scholars of every country pull out all the stops to study how to keep the original cultural features of literary works to the maximum extent while ensuring that foreign readers could fully understand and accept the content. Taking Chinese literature as an example, it always stands out as a shining pearl in world literature and is well known for its long history and rich types. Writings in classical Chinese like The Analects of Confucius, The Art of War and A Dream in Red Mansions are all concise in format but profound in significance. To better translate these literary works and promote Chinese culture, great efforts have been made at the academic level. While translating Evolution and Ethics, Yan Fu proposed the translation principle of “Faithfulness, Expressiveness and Elegance” which was regarded as the standard of translation (Yan & Zhu, 2017). Chinese renowned scholar Qian Zhongshu emphasized the consciousness of innovation and came up with “Sublimation Theory” in dealing with literary translation (Zheng, 2001).
.....
3.Research Design ................................................................................................. 16
3.1 Raw Materials .................................................................................................... 16
3.2 Linguistic Indicators .......................................................................................... 17
3.3 Research Tools ................................................................................................... 21
3.4 Summary ............................................................................................................ 24
4. Data Analysis and Results ................................................................................. 25
5. Case Studies ........................................................................................................ 31
5.1 Literary Text Cases and Machine Translations .................................................. 31
5.2 Non-literary Text Cases and Machine Translations ........................................... 39
5.3 Summary ............................................................................................................ 45
......
6. Discussions
6.1 Discussions on Corpus-driven Analysis Results
Generally, this corpus-driven study has two research approaches. First, the study calculated linguistic indicators values of chosen literary and non-literary texts and carried out the Pearson correlation analysis for each indicator to assess the machine translation performance. Second, on the basis of data analysis, altogether ten cases from both literary and non-literary texts were selected for detailed assessment of machine translation quality. Thus, discussions are given towards two approaches and corresponding results in the following two sections. The present study first collected data on three linguistic indicators of original texts (literature and non-literature) and their Google translations, and then computed the correlation coefficients of each pair (pair 1: literary text and its translation; pair 2: non-literary text and its translation). As shown in Table 6.1, the data demonstrate that, except the correlation analysis on Type Token Ratio (TTR) in which significant correlation exists in both pairs, the other two show similar results with a significant positive correlation existing between non-literary text and its machine translation and no significant correlation is found between literary text and its machine translation. The correlation existence provides the evidence that two variables are correlated with a very high statistical significance. In other words, there is a linear relationship between two variables. Google translation of non-literary text shares strong positive correlation with the source text in all three linguistic indicators in this study, indicating that machine translation performance on non-literary text is stable and consistent. By comparison, the result that only one significant correlation exists between the literary pair shows that machine translation performance on literary translation is basically not constant. The evaluation that machine translation performance on literary text is less stable and consistent than non-literary text is also supported by the standard deviations of the difference between the value of original text and that of machine translation for each indicator (see Table 6.2):
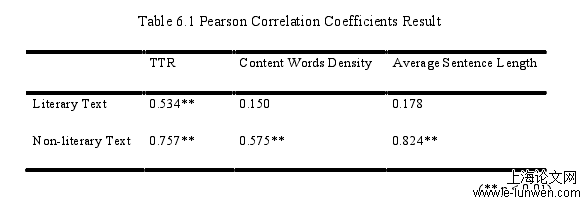
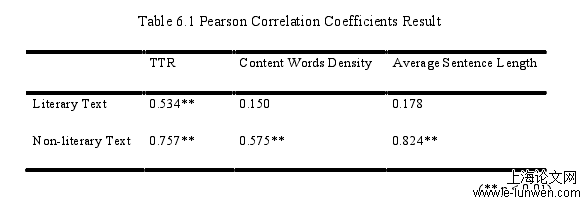
6.2 Discussions on Case Analysis Results
The changing trend of calculated three indicators values for each case is basically consistent with the overall sample text. For literary text, after being processed by machine, TTR, Average Sentence Length and Content Words Density values show a drop down trend, which means that the words richness, lexical density and sentence structure of original text are all simplified by machine translation. Besides, since there are many grammatical mistakes, misunderstandings and omissions in the machine translated texts, the translation quality is unsatisfactory and more technological upgrades are needed in helping machines comprehend culture-loaded words and different cultural contexts in literary works. By comparison, for non-literary text, though the words richness and lexical density values show a certain decline, the overall translation is accurate and smooth without information loss or misunderstanding, which provides users very good reading experience and is certainly able to meet the needs of machine translation users. In general, the final result that machine translation performance on literary text is barely satisfactory is in line with most people’s original judgement that the profound literary connotation can never be easily understood by machines. The most advanced translation machines make it possible to the batch processing of non-literary texts like legal documents, company files or news reports, but still fail to read between the lines when handling literary works. For better translating literary works, machine translation developers could consider collecting more training data on literature and designing translation systems more adaptive to literary narrative structure. There is a great demand for translating foreign literary works and it is expected that in the near future machine translation may offer support to lift translation efficiency and help promote foreign cultures.
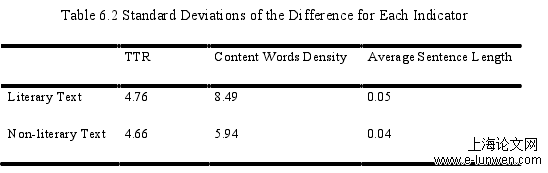
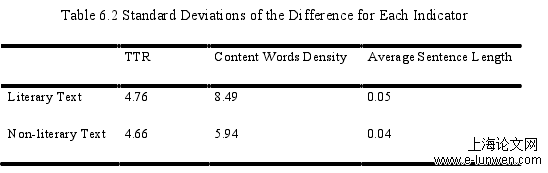
.....
7.Conclusions
(3) Is machine translation performance on literary translation equal to that of non-literary translation? Regarding the computational results of three linguistic indicators as well as case analysis result, the drawn conclusion is that machine translation of non-literary text outperforms the machine translation of literary text. And when dealing with literary text, machine translation has trouble figuring out the sentence structure and understanding specific culture-loaded words, as a result of which there are many mistransaltions and omssions in the output text. So far, the erratic performance of machine translation on literary text is unable to meet users’ expectations yet. Generally, it is best to bring more literature-related training data to the translation system and conduct more researches to help machine translation better understand literary works. The conclusion that machine translation of literary text still has a long way to go might disappoint some people while giving some others a break. However, the intension of conducting the research is to keep watch on the development of machine translation and update stakeholders by informing them of the latest process in machine translation field rather than create a sense of crisis. Only by knowing the degree of machine translation development can developers improve related techniques in a targeted direction and translators fully enjoy the convenience brought by technological progress.
参考文献(略)
参考文献(略)