参考文献的格式怎么写?本文以计算机论文为例,为大家列举80例论文的参考文献,希望能够帮助大家写好论文。
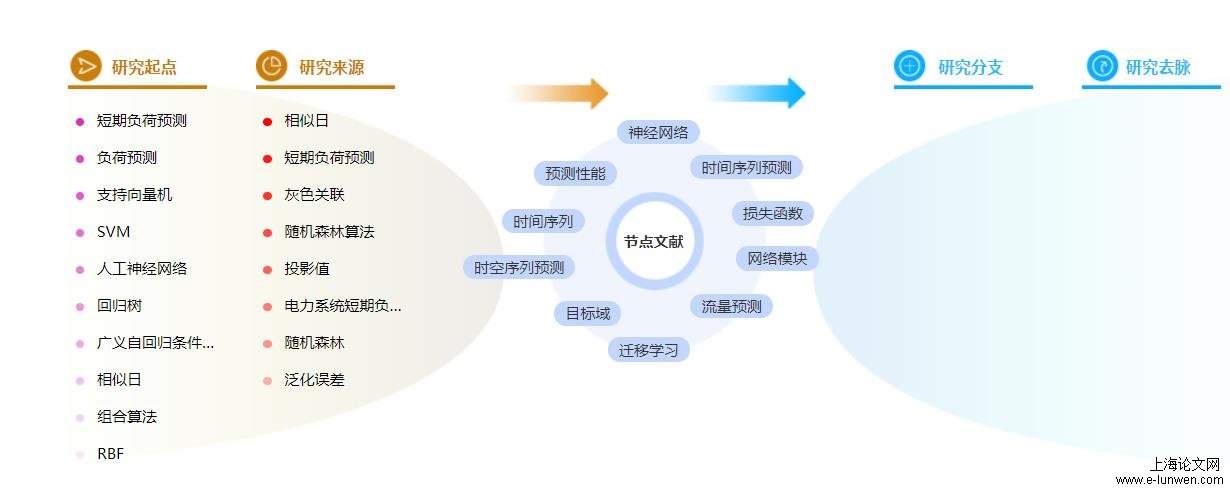
计算机论文参考文献
参考文献的格式案例一:神经网络在时间序列与时空序列流量预测中的应用与研究
[1]基于灰色投影改进随机森林算法的电力系统短期负荷预测[J]. 吴潇雨,和敬涵,张沛,胡骏. 电力系统自动化. 2015(12)
[1]A natural language-inspired multilabel video streaming source identification method based on deep neural networks[J] . Yan Shi,Dezhi Feng,Yu Cheng,Subir Biswas. Signal, Image and Video Processing . 2021 (prep)
[2]Deep multi-view residual attention network for crowd flows prediction[J] . Hao Yuan,Xinning Zhu,Zheng Hu,Chunhong Zhang. Neurocomputing . 2020 (prep)
[3]Short-term Load Forecasting of PowerSystem Based on Improved Feedforward Neural Network[J] . Wenbo Zhao,Xiaoqin Liu,Chengyu Li,Fengwei Zhang,Qian Wang. Journal of Physics Conference Series . 2020 (5)
[4]Citywide Traffic Flow Prediction Based on Multiple Gated Spatio-temporal Convolutional Neural Networks[J] . Cen Chen,Kenli Li,Sin G. Teo,Xiaofeng Zou,Keqin Li,Zeng Zeng. ACM Transactions on Knowledge Discovery from Data . 2020 (4)
[5]Traffic Flow Prediction at Varied Time Scales via Ensemble Empirical Mode Decomposition and Artificial Neural Network[J] . Xinqiang Chen,Jinquan Lu,Jiansen Zhao,Zhijian Qu,Yongsheng Yang,Jiangfeng Xian. Sustainability . 2020 (9)
[6]Citywide Cellular Traffic Prediction Based on a Hybrid Spatiotemporal Network[J] . Dehai Zhang,Linan Liu,Cheng Xie,Bing Yang,Qing Liu. Algorithms . 2020 (1)
[7]A Combined Deep-Learning and Transfer-Learning Approach for Supporting Social Influence Prediction[J] . Cuzzocrea Alfredo,Leung Carson K.,Deng Deyu,Mai Jiaxing Jason,Jiang Fan,Fadda Edoardo. Procedia Computer Science . 2020
[8]A Hybrid Deep Learning Model With Attention-Based Conv-LSTM Networks for Short-Term Traffic Flow Prediction[J] . Haifeng Zheng,Feng Lin,Xinxin Feng,Youjia Chen. IEEE Transactions on Intelligent Transportation Systems . 2020 (99)
[9]Using Fuzzy Uncertainty Quantization and Hybrid RNN-LSTM Deep Learning Model for Wind Turbine Power[J] . Lihua Lin,Min Li,Li Ma,Masooma Nazari,Sadegh Mahdavi,Arda Yunianta. IEEE Transactions on Industry Applications . 2020 (99)
[10]Multiple convolutional neural networks for multivariate time series prediction[J] . Kang Wang,Kenli Li,Liqian Zhou,Yikun Hu,Zhongyao Cheng,Jing Liu,Cen Chen. Neurocomputing . 2019 (C)
[11]Short-term load forecasting with clustering–regression model in distributed cluster[J] . Jingsheng Lei,Ting Jin,Jiawei Hao,Fengyong Li. Cluster Computing: The Journal of Networks, Software Tools and Applications . 2019 (10)
[12]Multi-scale dilated convolution of convolutional neural network for image denoising[J] . Yanjie Wang,Guodong Wang,Chenglizhao Chen,Zhenkuan Pan. Multimedia Tools and Applications . 2019 (14)
[13]Spatiotemporal traffic network analysis: technology and applications[J] . Huiyu Zhou,Kotaro Hirasawa. Knowledge and Information Systems . 2019 (1)
[14]Image super-resolution with densely connected convolutional networks[J] . Ping Kuang,Tingsong Ma,Ziwei Chen,Fan Li. Applied Intelligence . 2019 (1)
[15]A Spatial–Temporal Multitask Collaborative Learning Model for Multistep Traffic Flow Prediction[J] . Kun Tang,Shuyan Chen,Aemal J. Khattak. Transportation Research Record . 2018 (45)
[16]Demand Response Management in Smart Grid Networks: a Two-Stage Game-Theoretic Learning-Based Approach[J] . Apostolopoulos Pavlos Athanasios,Tsiropoulou Eirini Eleni,Papavassiliou Symeon. Mobile Networks and Applications . 2018 (prep)
[17]A multivariate short-term traffic flow forecasting method based on wavelet analysis and seasonal time series[J] . Zhang Hong,Wang Xiaoming,Cao Jie,Tang Minan,Guo Yirong. Applied Intelligence . 2018 (10)
[18]Deep Learning for Household Load Forecasting—A Novel Pooling Deep RNN[J] . Shi Heng,Xu Minghao,Li Ran. IEEE Transactions on Smart Grid . 2018 (5)
[19]Optimal Deep Learning LSTM Model for Electric Load Forecasting using Feature Selection and Genetic Algorithm: Comparison with Machine Learning Approaches ?[J] . Salah Bouktif,Ali Fiaz,Ali Ouni,Mohamed Adel Serhani. Energies . 2018 (7)
[20]A hybrid deep learning based traffic flow prediction method and its understanding[J] . Yuankai Wu,Huachun Tan,Lingqiao Qin,Bin Ran,Zhuxi Jiang. Transportation Research Part C . 2018
[21]A deep convolutional neural network to analyze position averaged convergent beam electron diffraction patterns[J] . W. Xu,J.M. LeBeau. Ultramicroscopy . 2018
[22]Integration of new evolutionary approach with artificial neural network for solving short term load forecast problem[J] . Priyanka Singh,Pragya Dwivedi. Applied Energy . 2018
[23]Recurrent Neural Networks for Multivariate Time Series with Missing Values.[J] . Che Zhengping,Purushotham Sanjay,Cho Kyunghyun,Sontag David,Liu Yan. Scientific reports . 2018 (1)
[24]A regional hybrid GOA-SVM model based on similar day approach for short-term load forecasting in Assam, India[J] . Mayur Barman,N.B. Dev Choudhury,Suman Sutradhar. Energy . 2018
[25]Short-Term Load Forecasting Using EMD-LSTM Neural Networks with a Xgboost Algorithm for Feature Importance Evaluation[J] . Huiting Zheng,Jiabin Yuan,Long Chen. Energies . 2017 (8)
[26]Short-term electrical load forecasting using the Support Vector Regression (SVR) model to calculate the demand response baseline for office buildings[J] . Yongbao Chen,Peng Xu,Yiyi Chu,Weilin Li,Yuntao Wu,Lizhou Ni,Yi Bao,Kun Wang. Applied Energy . 2017
[27]Short-term wind speed forecasting using the wavelet decomposition and AdaBoost technique in wind farm of East China[J] . Haijian Shao,Xing Deng,Fang Cui. IET Generation, Transmission & Distribution . 2016 (11)
[28]Traffic Flow Optimization on Freeways[J] . Bruno Carlo Rampinelli Rota,Milan Simic. Procedia Computer Science . 2016 (C)
[29]Electricity consumption modelling: A case of Germany[J] . Linh Phuong Catherine Do,Kuan-Heng Lin,Peter Molnár. Economic Modelling . 2016
[30]A multi-source dataset of urban life in the city of Milan and the Province of Trentino.[J] . Barlacchi Gianni,De Nadai Marco,Larcher Roberto,Casella Antonio,Chitic Cristiana,Torrisi Giovanni,Antonelli Fabrizio,Vespignani Alessandro,Pentland Alex,Lepri Bruno. Scientific data . 2015 (1)
[31]STARMA Models Estimation with Kalman Filter: The Case of Regional Bank Deposits[J] . Serkan Kurt,K. Batu Tunay. Procedia - Social and Behavioral Sciences . 2015
[32]Medium-Term Electric Load Forecasting Using Multivariable Linear and Non-Linear Regression[J] . Osama M. Aloquili,Fawwaz Elkarmi,Nazih Abu-Shikhah. Smart Grid and Renewable Energy . 2011 (2)
[33]Comparative models for electrical load forecasting | D.H. Bunn and E.D. Farmer, eds.(Wiley, New York, 1985) ?£24.95, pp. 232[J] . Sharp John. International Journal of Forecasting . 1986 (2)
[34]Neurons with graded response have collective computational properties like those of two-state neurons.[J] . Hopfield J J. Proceedings of the National Academy of Sciences of the United States of America . 1984 (10)
[1]Convolutional LSTM network:A machine learning approach for precipitation nowcasting. Xingjian SHI,Chen Z,Wang H et al. Advances in Neural Information Processing Systems . 2015
[2]Mobile Network Traffic Prediction Using MLP,MLPWD,and SVM. Nikravesh A Y,Ajila S,Lung C H. IEEE International Congress on Big Data . 2016
[3]Readings in Cognitive Science. Rumelhart,D.E,Hinton,G.E,Williams,R.J. MorganKaufmann . 1988
[4]Model-agnostic metalearning for fast adaptation of deep networks. FINN C,ABBEEL P,LEVINE S. Proceedings of the 34th International Conference on Machine Learning . 2017
[5]Short-term load forecasting using fuzzy logic and ANFIS. evik,H.H,unka,M. Neural Computing and Applications . 2015
[6]NB-CNN:deep learning-based crack detection using convolutional neural network and naive bayes data fusion. Chen F C,Jahanshahi M R. IEEE Transactions on Industrial Electronics . 2018
[7]Deep residual learning for image recognition. K.He,X.Zhang,S.Ren,et al. Computer Vision and Pattern Recognition . 2016
[8]Convolutional LSTM Network:a Machine Learning Approach for Precipitation Nowcast-ing. Shi Xingjian,Chen Zhourong,Wang Hao,et al. Neural Information Processing Systems 2015 . 2015
[9]A High Precision Artificial Neural Networks Model for Short-Term Energy Load Forecasting. Kuo P H,Huang C J. Energies . 2018
[10]City wide cellular traffic prediction based on densely connected convolutional neural networks. C. Zhang,H. Zhang,D. Yuan, et al. IEEE Communications Letters . 2018
[1]基于深度学习的城市时空序列预测模型及应用研究[D]. 杜圣东.西南交通大学 2020
[2]复杂环境下时间序列预测方法研究[D]. 刘海洋.北京交通大学 2019
[3]大规模多源时间序列预处理与隐藏空间映射分析研究[D]. 石巍巍.上海交通大学 2018
[4]心血管时间序列的图形分析和应用[D]. 颜昌.山东大学 2019
[5]面向超大规模时间序列的异常检测[D]. 黄田.上海交通大学 2015
[6]基于循环神经网络模型的遥感影像时间序列分类及变化检测方法研究[D]. 林蕾.中国科学院大学(中国科学院遥感与数字地球研究所) 2018
[7]时间序列流数据异常检测问题的研究[D]. 张琪.山东大学 2019
[8]局部相似分析的统计研究及其在生物时间序列中的应用[D]. 张方.山东大学 2019
[9]基于机器学习的时间序列模型研究及其应用[D]. 杨玉军.电子科技大学 2018
[10]随机交互金融模型的构建及金融时间序列的统计分析[D]. 鲁韵帆.北京交通大学 2018
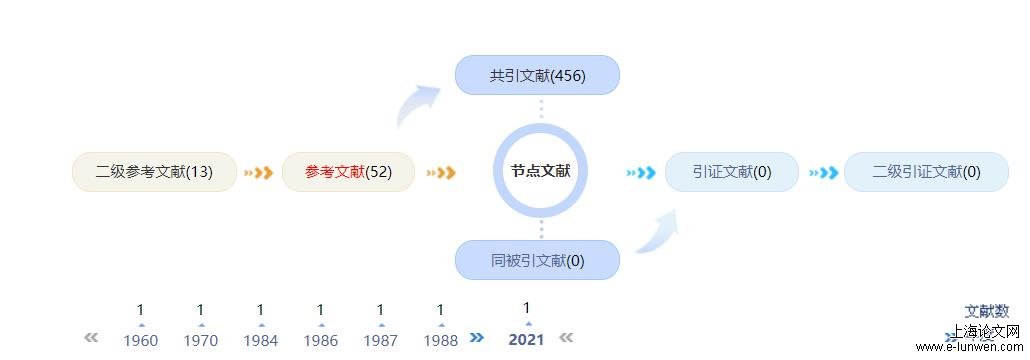
博士论文参考文献
参考文献的格式案例二:基于高速荧光透视成像的运动鞋帮高对足踝侧切动作影响的生物力学研究
[1]不同鞋帮类型足球鞋对踝关节稳定性的影响[J]. 魏书涛. 皮革科学与工程. 2021(01)
[2]双平面正交荧光透视成像系统在运动医学领域中的应用研究进展[J]. 张翠,汤运启,王少白,刘宇. 中国运动医学杂志. 2019(08)
[3]4种算法评估落地动作中ACL负荷的对比研究[J]. 黄尚军,伍勰,阮棉芳,张强,陈晨. 体育科学. 2018(03)
[4]女篮运动员下肢损伤情况同赤脚与穿鞋状态下最大翻转力矩差别高度相关(英文)[J]. Jennifer M.Yentes,Max J.Kurz,Nicholas Stergiou. Journal of Sport and Health Science. 2014(03)
[5]外踝骨折后三角韧带损伤程度的X线与MRI比较研究[J]. 王淑丽,马信龙,徐卫国,潘涛,张晓光,崔壮. 中华骨科杂志. 2013 (08)
[6]高、低帮篮球鞋对落地时踝关节稳定及肌肉功能影响的研究[J]. 侯建甫,魏春生,傅维杰,魏书涛,何俊良,刘宇. 体育科学. 2011(05)
[7]踝关节侧副韧带损伤超声检查[J]. 林发俭,冉维强,黄曼维,王金锐. 中国医学影像技术. 2002(12)
[1]高冲击动作中在体胫股关节6自由度运动和软骨接触特征研究[D]. 张翠.上海体育学院 2020
[2]疲劳与非预期因素对女子足球运动员变向动作中下肢生物力学的影响[D]. 邹利民.上海体育学院 2019
[3]功能性踝关节不稳者动态姿势稳定性评价方法及其影响因素的研究[D]. 尹彦.北京体育大学 2016
[4]距骨数值仿真模型的建立及有限元分析[D]. 卢昌怀.南方医科大学 2011
[1]不同防护方式对慢性踝关节不稳人群步态启动及平衡功能的即刻影响[D]. 时旺然.上海体育学院 2019
[2]足-鞋有限元模型的建立及在足底筋膜研究中的应用[D]. 刘姣姣.北京体育大学 2019
[3]运动鞋后跟高度对上坡行走步态的生物力学特征影响[D]. 蒋量.北京体育大学 2018
[4]踝关节内侧韧带解剖学与影像学及生物力学研究[D]. 谢龙.南方医科大学 2015
[5]足踝生物力学研究及有限元模型的建立[D]. 张伟.南方医科大学 2012
[6]高低帮篮球鞋对跳跃动作下肢的生物力学影响[D]. 何俊良.上海体育学院 2011
[7]高低帮篮球鞋对下肢生物力学特征的影响[D]. 程鹏.北京体育大学 2010
[1]Stability of the loaded ankle. Relation between articular restraint and primary and secondary static restraints. Stormont D M,Morrey B F,An K N,Cass J R. The American journal of sports medicine . 1985
[2]Effects of circumferential ankle pressure on ankle proprioception, stiffness, and postural stability: a preliminary investigation. You Sung H,Granata Kevin P,Bunker Linda K. The Journal of orthopaedic and sports physical therapy . 2004
[3]高低帮篮球鞋对下肢生物力学特征的影响. 程鹏. 北京体育大学 . 2010
[4]Estimation of ligament strains and joint moments in the ankle during a supination sprain injury. Wei F,Fong D T-P,Chan K-M,et al. Computer Methods . 2013
[5]A biomechanical study of human lateral ankle ligaments and autogenous reconstructive grafts. Attarian D E,McCrackin H J,Devito D P,McElhaney J H,Garrett W E. The American journal of sports medicine . 1985
[6]不同防护方式对慢性踝关节不稳人群步态启动及平衡功能的即刻影响. 时旺然. 上海体育学院 . 2019
[7]距骨数值仿真模型的建立及有限元分析. 卢昌怀. 南方医科大学 . 2011
以上是80例计算机论文的参考文献,如果你想了解更多关于论文写作的素材或者方法,可以在本网站查询;如果需要寻求写作帮助,可以在线咨询。